Video captioning fashions are generally educated on datasets consisting of quick movies, normally underneath 3 mins in period, paired with corresponding captions. Whilst this allows them to explain elementary movements like strolling or speaking, those fashions battle with the complexity of long-form movies, corresponding to vlogs, sports activities occasions, and films that may remaining over an hour. When carried out to such movies, they incessantly generate fragmented descriptions keen on remoted movements somewhat than shooting the wider storyline. Efforts like MA-LMM and LaViLa have prolonged video captioning to 10-minute clips the usage of LLMs, however hour-long movies stay a problem because of a scarcity of appropriate datasets. Despite the fact that Ego4D offered a big dataset of hour-long movies, its first-person viewpoint limits its broader applicability. Video ReCap addressed this hole through coaching on hour-long movies with multi-granularity annotations, but this method is pricey and at risk of annotation inconsistencies. Against this, annotated short-form video datasets are extensively to be had and extra user-friendly.
Developments in visual-language fashions have considerably enhanced the mixing of imaginative and prescient and language duties, with early works corresponding to CLIP and ALIGN laying the basis. Next fashions, corresponding to LLaVA and MiniGPT-4, prolonged those features to pictures, whilst others tailored them for video working out through that specialize in temporal series modeling and establishing extra tough datasets. Regardless of those traits, the shortage of huge, annotated long-form video datasets stays an important hindrance to growth. Conventional short-form video duties, like video query answering, captioning, and grounding, essentially require spatial or temporal working out, while summarizing hour-long movies calls for figuring out key frames amidst really extensive redundancy. Whilst some fashions, corresponding to LongVA and LLaVA-Video, can carry out VQA on lengthy movies, they fight with summarization duties because of information obstacles.
Researchers from Queen Mary College and Spotify introduce ViSMaP, an unmanaged approach for summarising hour-long movies with out requiring pricey annotations. Conventional fashions carry out neatly on quick, pre-segmented movies however battle with longer content material the place essential occasions are scattered. ViSMaP bridges this hole through the usage of LLMs and a meta-prompting solution to iteratively generate and refine pseudo-summaries from clip descriptions created through short-form video fashions. The method comes to 3 LLMs operating in series for era, analysis, and recommended optimisation. ViSMaP achieves efficiency similar to totally supervised fashions throughout more than one datasets whilst keeping up area adaptability and getting rid of the will for in depth guide labelling.
The learn about addresses cross-domain video summarization through coaching on a labelled short-form video dataset and adapting to unlabelled, hour-long movies from a distinct area. First of all, a style is educated to summarize 3-minute movies the usage of TimeSFormer options, a visual-language alignment module, and a textual content decoder, optimized through cross-entropy and contrastive losses. To maintain longer movies, they’re segmented into 3-minute clips, and pseudo-captions are generated. An iterative meta-prompting method with more than one LLMs (generator, evaluator, optimizer) refines summaries. In spite of everything, the style is fine-tuned on those pseudo-summaries the usage of a symmetric cross-entropy loss to control noisy labels and enhance adaptation.
The learn about evaluates VisMaP throughout 3 eventualities: summarization of lengthy movies the usage of Ego4D-HCap, cross-domain generalization on MSRVTT, MSVD, and YouCook2 datasets, and adaptation to quick movies the usage of EgoSchema. VisMaP, educated on hour-long movies, is when put next towards supervised and zero-shot strategies, corresponding to Video ReCap and LaViLa+GPT3.5, demonstrating aggressive or awesome efficiency with out supervision. Critiques use CIDEr, ROUGE-L, METEOR rankings, and QA accuracy. Ablation research spotlight some great benefits of meta-prompting and part modules, corresponding to contrastive finding out and SCE loss. Implementation main points come with using TimeSformer, DistilBERT, and GPT-2, with coaching carried out on an NVIDIA A100 GPU.
In conclusion, ViSMaP is an unmanaged method for summarizing lengthy movies through the use of annotated short-video datasets and a meta-prompting technique. It first creates top of the range summaries thru meta-prompting after which trains a summarization style, decreasing the will for in depth annotations. Experimental effects show that ViSMaP plays on par with totally supervised strategies and adapts successfully throughout more than a few video datasets. Alternatively, its reliance on pseudo labels from a source-domain style would possibly affect efficiency underneath vital area shifts. Moreover, ViSMaP recently is predicated only on visible knowledge. Long run paintings may combine multimodal information, introduce hierarchical summarization, and expand extra generalizable meta-prompting ways.
Take a look at the Paper. Additionally, don’t disregard to apply us on Twitter and sign up for our Telegram Channel and LinkedIn Group. Don’t Overlook to sign up for our 90k+ ML SubReddit.
🔥 [Register Now] miniCON Virtual Conference on AGENTIC AI: FREE REGISTRATION + Certificate of Attendance + 4 Hour Short Event (May 21, 9 am- 1 pm PST) + Hands on Workshop
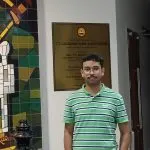
Sana Hassan, a consulting intern at Marktechpost and dual-degree scholar at IIT Madras, is making use of era and AI to handle real-world demanding situations. With a willing pastime in fixing sensible issues, he brings a contemporary viewpoint to the intersection of AI and real-life answers.
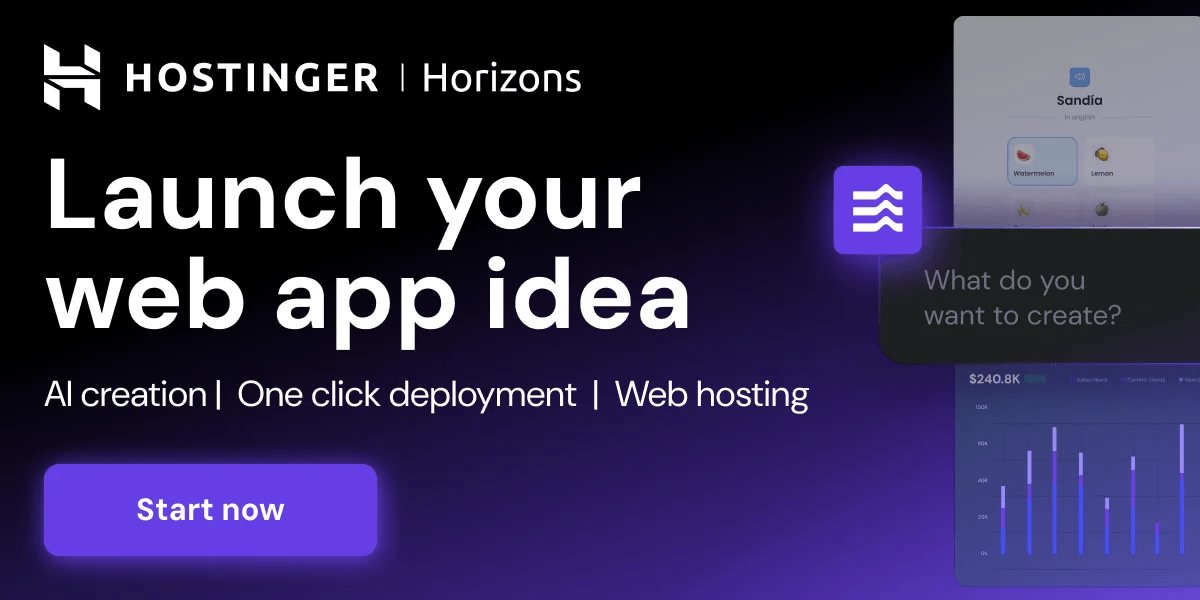
Source link